Generative AI: Platforms, Network Effects, and the Economics of Abundance
Generative AI is all the rage these days. You can hardly go a day without stumbling across a new development in the field or think pieces prescribing what the future of AI holds. But how do network effects tie in? In our view, generative AI companies don’t have network effects of their own (at least so far), although they could have an impact on networks in adjacent markets.
Sameer Singh is the creator of breadcrumb.vc, a blog providing investors and founders with resources on all things network effects. He’s also the founder of the Applied Network Effects course, one of the highest-rated courses on Maven, and an Atomico Angel who exclusively invests in Pre-Seed and Seed-stage startups with network effects.
We’ve previously turned to Sameer for his thoughts on whether tokens can solve the cold start problem. He has also been a recurring contributor to our Marketplace Conference and shares several portfolio companies with us, such as Lapse. With that in mind, it shouldn’t come as a surprise that he’s joining our Marketplaces & Consumer team as a Venture Partner now! So it was the perfect time to welcome him to the team and bring him back to share his thoughts on generative AI and the importance of authentic content.
Generative AI: Platforms, Network Effects and the Economics of Abundance
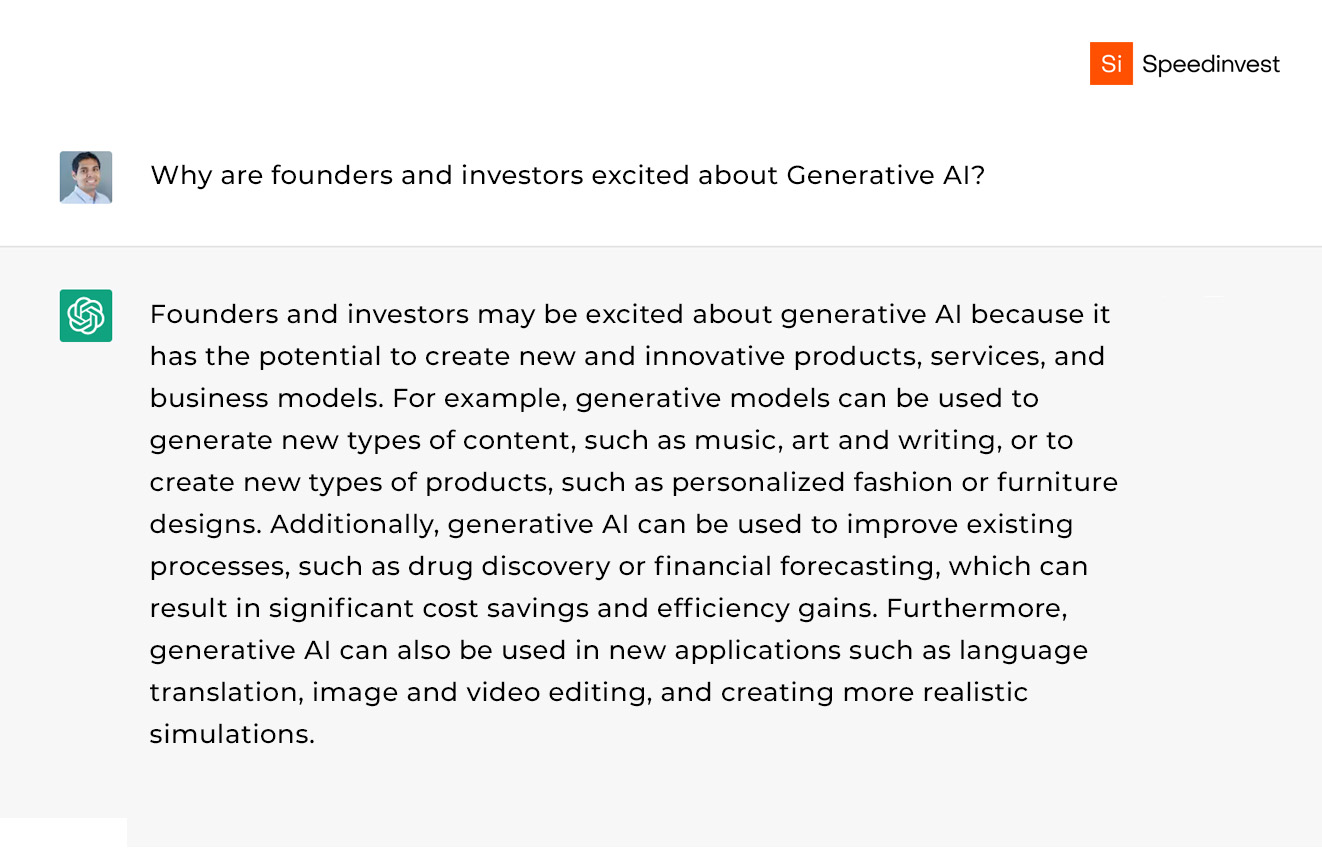
Generative AI is clearly having a moment. While AI models in this space have been on a sustained trajectory of improvement for the last few years, 2022 seemed to be an inflection point. Throughout 2022, we saw the launch of AI image generators like Midjourney, Stability’s Stable Diffusion, OpenAI’s DALL-E 2 and, of course, OpenAI’s chatbot ChatGPT — powered by its large language model (LLM), GPT-3.
There’s a clear sense that these launches represent a step change in AI capabilities. This has led to a lot of excitement from founders and investors—and speculation about the potential of AI platform businesses and AI-generated content networks, i.e. new iterations of network effects for the “AI era.” Let’s take a deeper look at the reality behind the hype.
Before I go any further, let me give some context by defining the term “Generative AI.” It refers to AI models that can create content including blog posts, email copy, images, video, and even code from simple text prompts. Unlike the models designed for, say, autonomous vehicles, the output here is useful even if it’s 80–90 percent accurate (maybe even less). Humans may need to improve this AI-generated output before deploying it, but it still dramatically cuts the time and cost of creating content. In other words, the utility of these advances is not in question.
I’ll stop here. There are many people more qualified to explain potential use cases of Generative AI. Instead, let me go back to the point of this post — are AI platform businesses and AI content networks a thing?
Platform Network Effects?
First, let’s address the AI platform business. I’ve previously explained that you need four components to create a platform—an underlying product, a development framework, matching, and an economic benefit. Deep learning models from the likes of OpenAI only meet one of these criteria: they allow developers to leverage these models to create new applications (development framework). Crucially, they do not have an underlying product and so don’t own end-users.
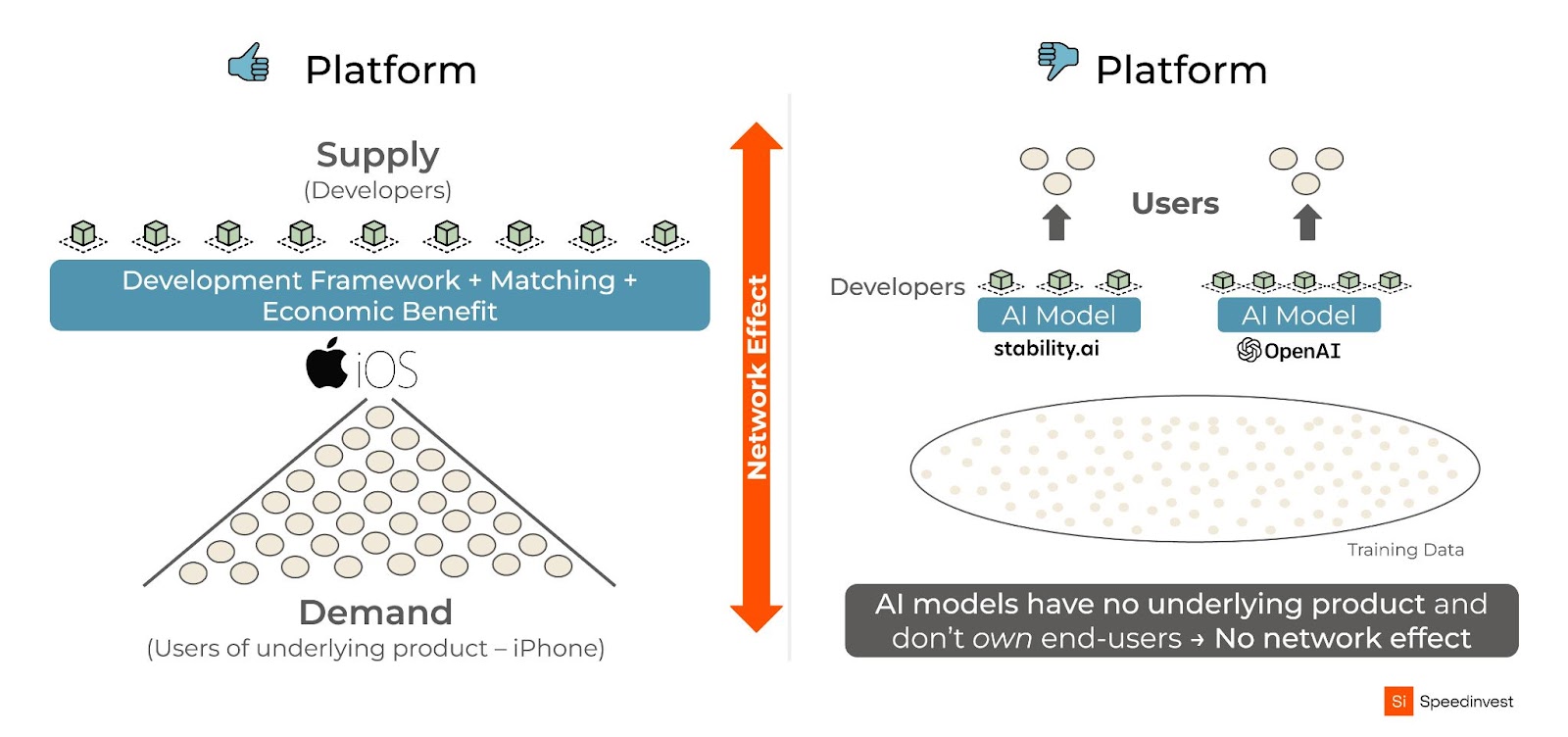
Why do platforms need an underlying product in the first place? An underlying product (e.g. the iPhone for iOS) enables a network effect between the users of the product on one side and third-party developers building apps on top of that product on the other, i.e. the addition of developers makes the underlying product more valuable for all users—a platform network effect. Without an underlying product, there is no possibility of a network effect.
The choice of a specific generative AI model has virtually no impact on end-users. Why would users care if an AI app is built on DALL-E 2 or Stable Diffusion? Or if it uses Google’s LaMDA instead of GPT-3? Early movers like OpenAI may have a near-term technical advantage, i.e. there’s a possibility that their models may be more advanced than competitors for the time being. Or they might benefit from embedding themselves into developers’ workflows. Or the cultural impact of ChatGPT’s rapid growth may lead to brand loyalty. But none of these advantages creates a platform network effect.
What about data network effects? Do foundational models like GPT-3 or Stable Diffusion benefit from those? Here again, the answer is no. Data network effects also require four components––proprietary data, a feedback loop, data ownership, and a link to the core value proposition.
Proprietary data is at the heart of this. But foundational AI models have been trained on publicly available data (not proprietary). Users do not create the data these models are trained on. This means that they do not have a feedback loop and so they cannot have any data network effects. And since they don’t own the end user, they have limited avenues to create proprietary data.
Over time, there may be situations where the owner of a foundational model (e.g. OpenAI) creates an end-user application (e.g. ChatGPT) that collects proprietary data from users to improve the model. This could create avenues for some data network effects down the line.
Applications: Content Networks?
Next, let’s address AI-generated content “apps” (note: apps, not networks). Content networks, like TikTok allow users to create content for other users, i.e. the addition of a creator increases the value of the product for other users — another network effect (what I would call an interaction network).
You could argue that TikTok’s network effects have weak defensibility (as I have), because the identity of the content creator is not central to its value proposition, i.e. its users are commoditized, unlike Snapchat or WhatsApp users. This makes it easier for viable alternatives to exist — including Snapchat Spotlight, Instagram Reels and YouTube shorts. However, it still receives some benefits from its network effects — competing networks need to meet the threshold of a “good enough” base of creators to create a viable alternative. This is not true for AI-generated content “apps”.
Lensa AI and Frivolous Apps
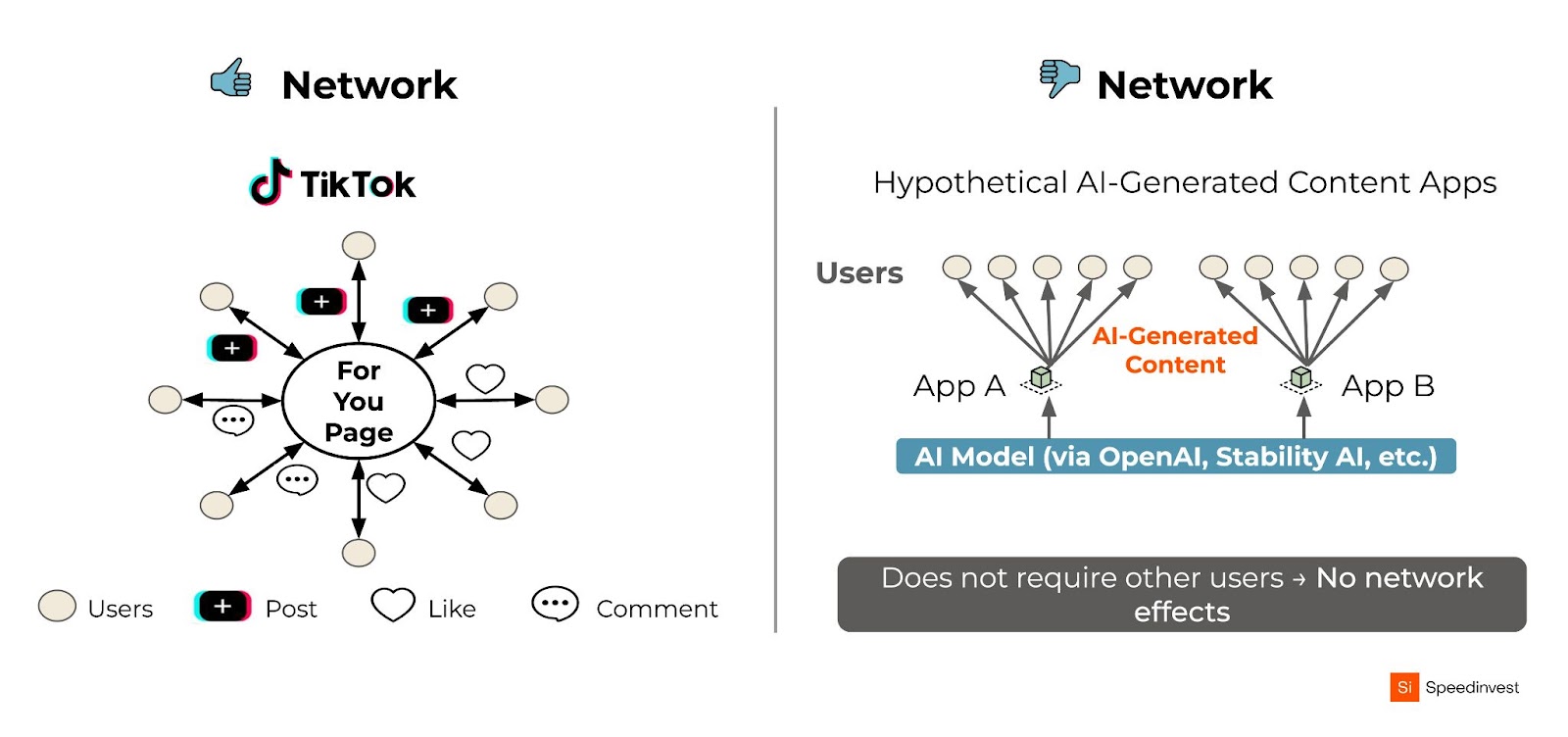
Anyone with sufficient coding and design knowledge can leverage off-the-shelf models to entertain users with AI-generated content. This might seem like a stretch, but we have already seen this happen. For example, Lensa AI’s avatar generator, built on Stable Diffusion, went viral in December 2022 and was able to generate millions of dollars in revenue over a period of a few weeks.
However, its popularity led to a whole sea of clones, including “Dawn AI”, “AI Art”, “Wonder AI”, and so on. A remarkably quick journey from novel product to commoditization — comparable to the competition between frivolous apps in the early days of the iOS app store, including iBeer, lightsaber apps, and so on.

This structural characteristic does not change even if/when the AI models powering these content apps become advanced enough to generate TikTok-quality content. They can just as easily be leveraged by the next app developer. Products that are commoditized from day 0 (or day 1 if we’re being generous) are unlikely to ever become large enough to matter.
Economics of Abundance
The utility of generative AI to end users is abundantly clear. What’s less clear is the type of companies that become more valuable because of it. Hardware and infrastructure companies are good candidates — as the a16z team eloquently described in this essay. But what about businesses with network effects? Is generative AI likely to have any impact there?
The short answer is yes — especially, if we keep an eye on second order effects. What happens when high quality content can be created with a click of a button and at minimal cost? Does this change what users value? To answer this question, we need to understand the economics of abundance. Here’s a summary of the concept from an essay I wrote about mobile gaming back in 2014:
“Economics is essentially a social science that examines the best possible way to allocate’“scarce’ goods or resources, i.e. ones with meaningful marginal cost and limited supply. However, digital goods like apps are abundant because the marginal cost of creating an additional copy is zero. Given the nature of near-efficient competition in the digital world, price naturally approaches the marginal cost of zero.
This explains the decline in popularity of paid app downloads and the decline of numerous traditional business models. However, cheap or free content allows developers to reach a much wider audience which consequently increases demand for related scarce goods or resources. In the music industry, the advent of digital music precipitated a steep decline in US recorded music sales from $14.6 billion in 1999 to just $6.3 billion in 2009, but concert ticket sales grew from $1.5 billion to $4.6 billion over the same timeframe. In other words, digital music converted a scarce resource (recorded music albums) into an abundant resource (cheap, easily downloadable singles), which then increased demand for a related scarce resource, i.e. concert tickets.”
It’s remarkable how much of this directly applies to generative AI. If zero (or low) marginal cost content becomes abundant, the value of that content drops to near-zero. What related scarcities does this create?

A few candidates are “authentic content” based on real-world relationships and experiences — and there could be some overlap between these areas.
- “Authentic content” is created by someone you have followed and built up a relationship with over time, i.e. not just “entertaining content.” In a sea of same-ness, authentic content based on genuine fandom carries more value. And of course, this also increases demand for apps that check the "authenticity" of content (to ensure that it is not AI-generated).
- Real-world relationships and experiences also become more scarce on a relative basis. Any content from or interactions with people you know become more valuable compared to the enormous amount of generated content that you are likely to come across. Similarly, live sports, arts, culture, travel, and so on all become more appealing because they are real.
Networks built on these atomic units have the potential to break out alongside generative AI and among early-stage networks in Europe, Howbout and Lapse are two obvious companies that come to mind. Of course, my imagination is limited. This is where founders come in. I suspect the most exciting forms of network effects might just be the ones that I couldn’t possibly imagine right now.
What you should do next
Pitch us: No warm intros required
We invest together in pre-Seed and Seed startups: Sameer via the Atomico Angel Program and Speedinvest from our latest €300M+ flagship seed fund.
Apply for my cohort-based course
Want to learn more about network effects? I run a 3-week long, workshop-style course on building, evaluating, scaling, and monetizing network effects. Register now to secure your place.
Sign up for email updates with the Marketplace Snippet
The Marketplace Snippet is a monthly newsletter created and curated by the Marketplaces & Consumer team at Speedinvest, including updates on the annual Marketplace Conference and year-round meetups. Sign up here.
Want more updates on our portfolio? Sign up for our monthly newsletter and follow us on LinkedIn.